LDW DataThinks: Building Better AI in the Open
Throughout this month, to celebrate London Data Week, LOTI will be publishing a series of think-pieces, ‘DataThinks’, written by experts working with data and artificial intelligence (AI), challenging data practitioners in London local government and beyond to think about data in new ways. The views expressed within each article are solely those of the authors.
This article is written by Jennifer Ding,TPS Senior Researcher – Research Applications at The Alan Turing Institute.
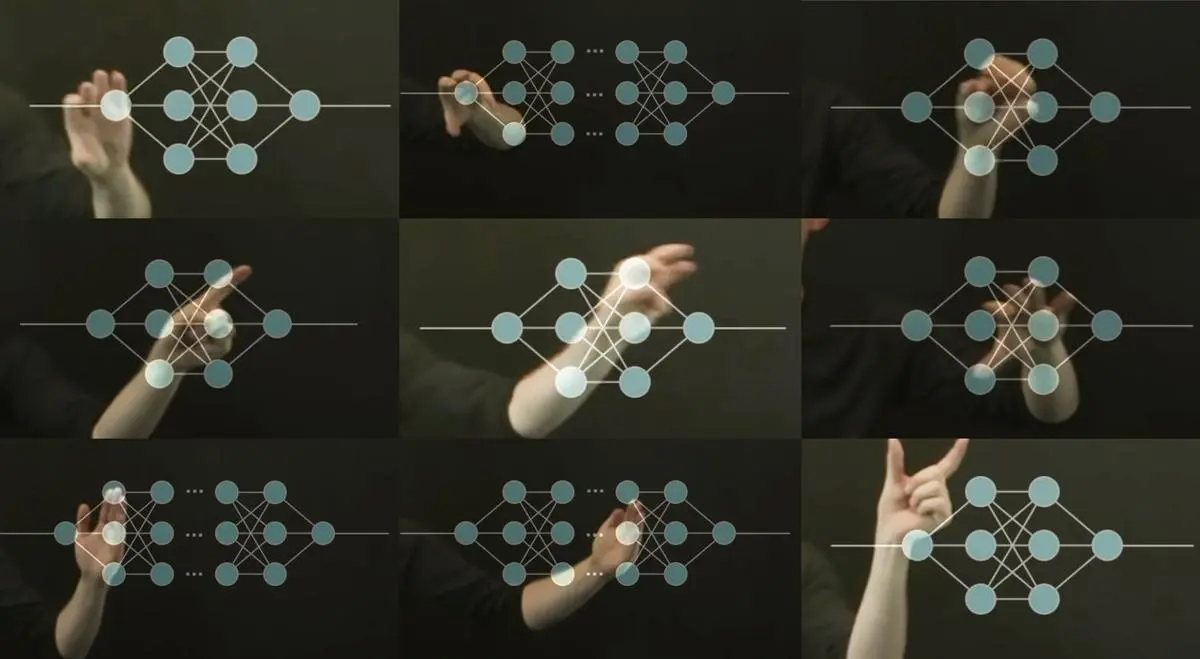
Alexa Steinbrück / Better Images of AI / Explainable AI / CC-BY 4.0
Drawing inspiration from the open source software, open science, and open data movements, open source AI has enabled alternative pathways to emerge for how AI is produced and who can be part of the process and shape the values embedded in the technology. But how do we define the “open” in “open source AI”? In AI, does openness refer to access to the artefacts of machine learning, such as the data, trained weights, and model outputs, or to the process of production and governance? The latest AI advancements have complicated our understanding of many aspects of technology and society, including what new outcomes openness brings.
As one of the co-founders of London Data Week, a citywide festival centring the public in events around data and AI, I wanted to take the opportunity this year to examine these pressing questions with our community. Through conversations with AI experts like Margaret Mitchell, Chief Ethics Scientist at Hugging Face, and creative workshops with organisations like Better Images of AI to better understand and depict what Large Language Models (LLMs) can bring to local government, we immersed ourselves in this question: how can we build AI that is better for more people, and how can open source AI support that process?
- Open source AI enables faster innovation and better performance
Open source AI models like Stable Diffusion and Falcon have been shown to match or beat performance of their closed counterparts like DALLE-2 and GPT, challenging longheld beliefs that state-of-the-art models can only be produced by a handful of technology companies in even fewer countries. Even Google has noted how open source AI developers, distributed around the world, are proving that smaller, fine-tuned models can outperform larger models, or in their words “eating our lunch.”
Open source AI is upending the misconception that “bigger is better” and that in order to build state-of-the-art one needs Internet-size datasets, even deeper architectures, and ever longer training times. This phenomenon, embodied by the flurry of development sparked by the leaking of Meta’s LLaMA but also characteristic of past open releases for models like YOLO and BERT, demonstrates the pace and scale of innovation in the open source AI community. At London Data Week’s kickoff event, Margaret Mitchell expanded on these opportunities and how open source can be an antidote to reducing the AI hype while also empowering more people and businesses to develop meaningful new AI applications.
- Open source AI enables more expansive and inclusive definitions of “better” AI
Beyond the performance metrics the AI world uses to compare and rank models, open source AI introduces new ways of understanding what “better” AI looks like and what characteristics make a model worthy of public attention and use. AI ethics experts have questioned over the years whether models that are biased against certain groups or require enormous expenditures of energy to train among other social harms should be considered “good enough”. During London Data Week, we invite Londoners from different backgrounds to create better images of AI that align with their values and challenge what meaningful public participation with AI labs looks like, such as connecting the practice of open documentation of datasets and models to auditable actions to demonstrate accountability.
- Open source AI enables more equitable and empowering data practices
In recognition of data quality (into and out of a model) as one of the crucial components required for better AI, many London Data Week events also focus on exploring these issues, such as our events with The Francis Crick Institute and Profusion on the data equity gaps in genome editing and women’s healthcare. Other events focus on encouraging Londoners to collect and visualise their own data to address topics of their interest. Openness provides the environment in which these core ingredients like identifying and addressing data biases and building awareness and ownership over our own data, can enable people to understand and engage with AI in more tangible and impactful ways. This includes creating governance mechanisms to empower data creators like artists to achieve better outcomes for “consent, credit, and compensation.”
By challenging the status quo and expanding participation to invite artists, civil servants, philosophers, students, and more people into the conversation about and production of AI, open source AI leads to more sustainable, more beneficial, and better performing models by the definitions of more people. Through open source AI, we see a practice of openness that goes beyond expanding access. We see openness for faster innovation, for broadening participation, and as a response to addressing power imbalance, so that more people can be part of and benefit from an AI future. London Data Week is inspired by similar values of fostering data & AI in the public, for the public and we look forward to expanding this conversation in London this week and with more people around the world in the future.
To find out more about London Data Week and the events taking place, visit the website.
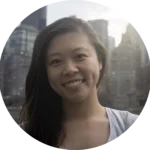
Jennifer Ding